These days I like to discuss
- Analytic Design Theory
- Statistical Communication
- The Casual Inference Podcast
- Large-scale medical data
- Italian
- Co-founding R-Ladies Nashville
- Disney World
over coffee
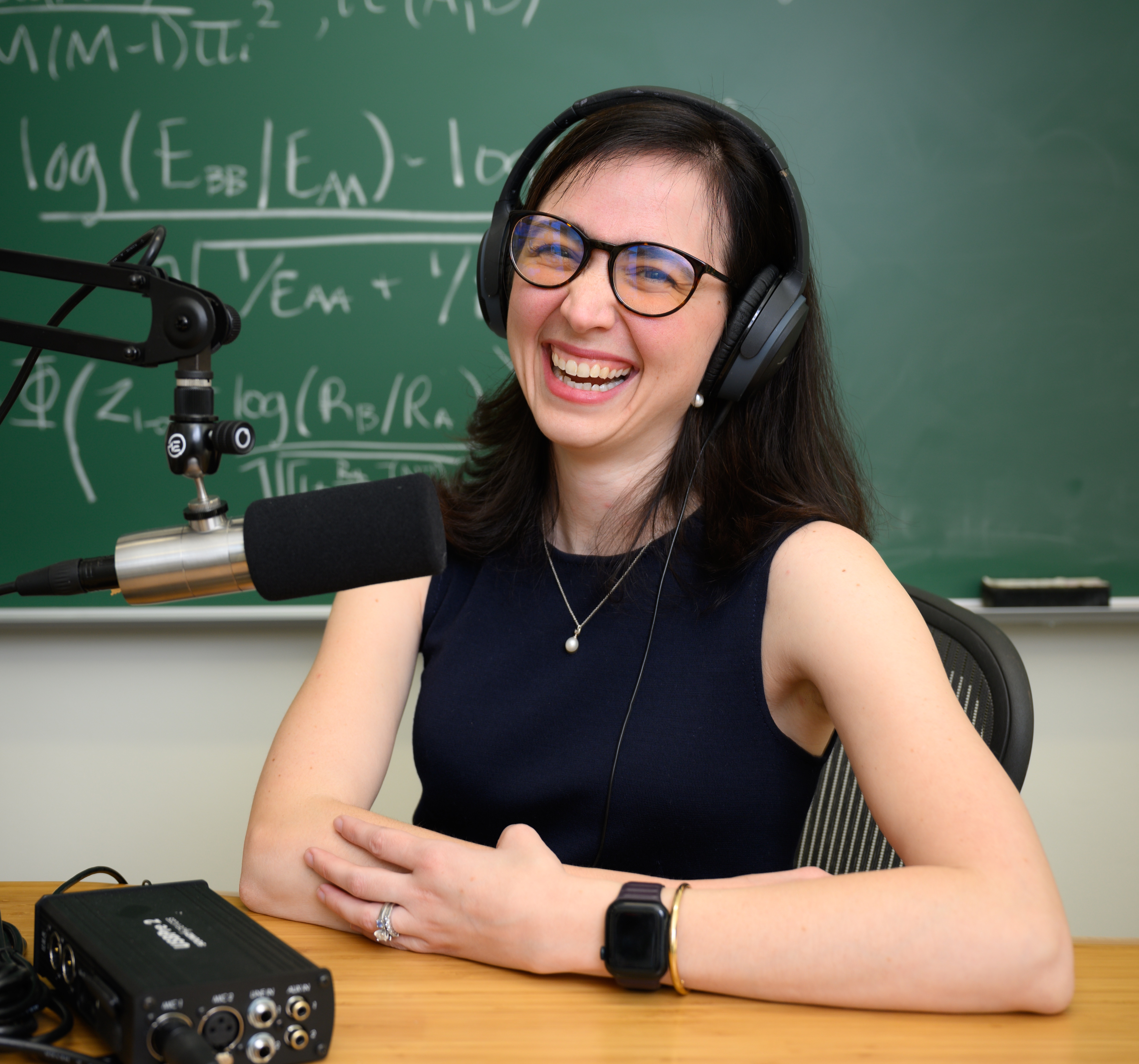
Lucy D’Agostino McGowan
Lucy D’Agostino McGowan is an assistant professor in the Department of Statistical Sciences at Wake Forest University. She received her PhD in Biostatistics from Vanderbilt University and completed her postdoctoral training at Johns Hopkins University Bloomberg School of Public Health. Her research focuses on causal inference, statistical communication, analytic design theory, and data science pedagogy. Dr. D’Agostino McGowan was the 2023 chair of the American Statistical Association’s Section on Statistical Graphics and can be found blogging at livefreeordichotomize.com, on Twitter @LucyStats, and podcasting on the American Journal of Epidemiology partner podcast, Casual Inference.
Recent Awards
- In 2025, Lucy received the Emerging Leader Award from the Committee of Presidents of Statistical Societies
- In 2023, Lucy was selected for the Teaching in the Health Sciences Young Investigator Award for her paper Design Principles for Data Analysis
- In 2023, Lucy was selected as an ASA StatsForward Fellow
Listen to the Casual Inference Podcast
Recent & Upcoming Talks
Causal Inference in R
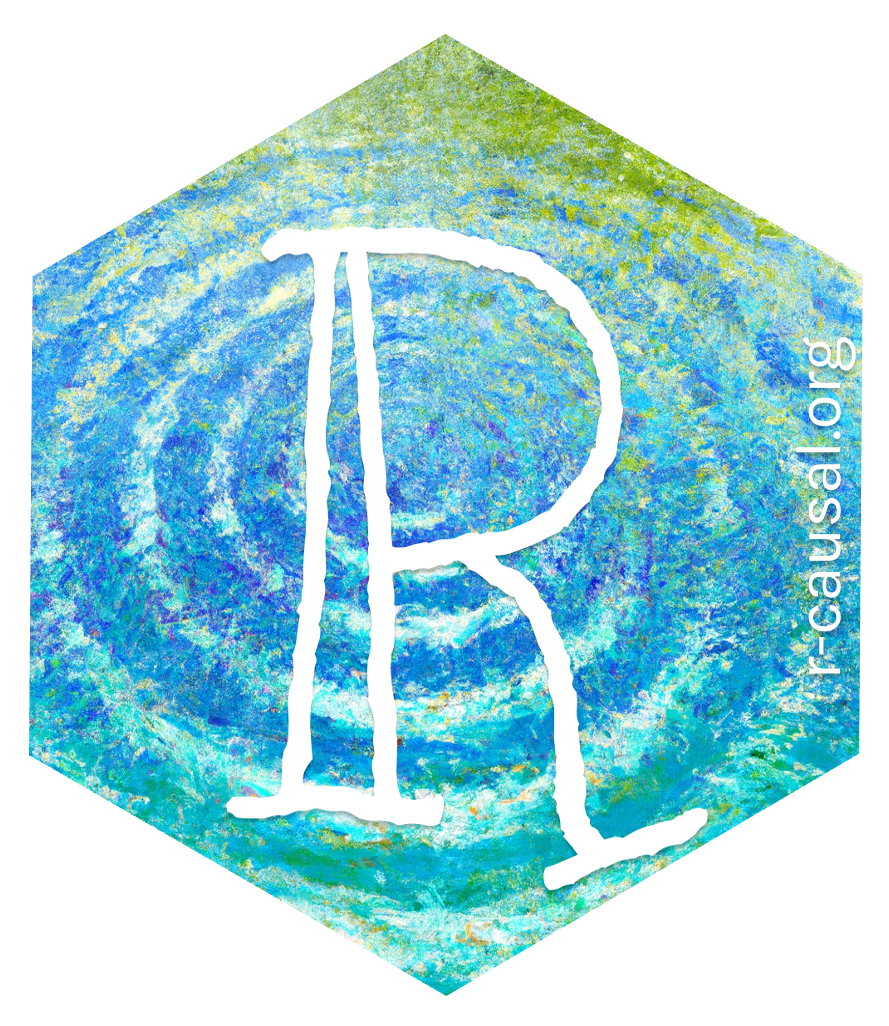
In this workshop, we’ll teach the essential elements of answering causal questions in R through causal diagrams, and causal modeling techniques such as propensity scores and inverse probability weighting. In both data science and academic research, prediction modeling is often not enough; to answer many questions, we need to approach them causally. In this workshop, we’ll teach the essential elements of answering causal questions in R through causal diagrams, and causal modeling techniques such as propensity scores and inverse probability weighting. We’ll also show that by distinguishing predictive models from causal models, we can better take advantage of both tools. You’ll be able to use the tools you already know–the tidyverse, regression models, and more–to answer the questions that are important to your work. This workshop is for you if you: know how to fit a linear regression model in R, have a basic understanding of data manipulation and visualization using tidyverse tools, and are interested in understanding the fundamentals behind how to move from estimating correlations to causal relationships.
Read moreUnderstanding Statistics in Medical Literature

In today’s fast-paced healthcare landscape, understanding data and statistics is essential for making informed decisions. Whether you’re a medical student navigating your first journal article or a healthcare professional hoping to apply the latest research to patient care, the ability to critically evaluate medical literature is a vital skill. This course is designed to introduce you to the core concepts of data and statistics, equipping you with the tools to extract meaningful insights from research without becoming bogged down in complex mathematical notation.
Read moreThe Case for Deterministic Imputation in Predictive Modeling
While multiple imputation is widely accepted for handling missing data in clinical research, its default use in predictive modeling may be inappropriate. Multiple imputation relies on access to the outcome variable to avoid bias, an assumption that breaks down in real-world deployment where the outcome is unknown. This talk argues that deterministic imputation methods, which do not depend on the outcome and are computationally efficient, are better suited for building predictive models intended for deployment. We present theoretical results and simulation evidence demonstrating that deterministic imputation maintains model validity and performance without introducing information leakage. We conclude that for predictive tasks, particularly in clinical settings where transparency, reproducibility, and alignment with deployment conditions are essential, deterministic imputation should be the standard.
Read moreTeaching
STA 112 -- WFU Spring 2024
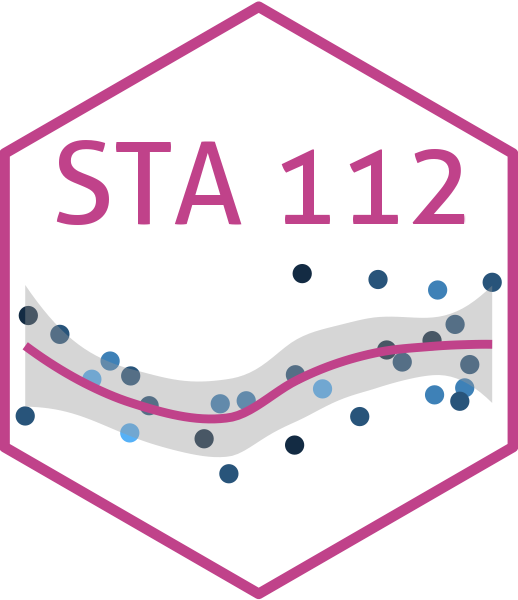
Introduction to Regression and Data Science. Learn to explore, visualize, model, evaluate, and communicate data in a reproducible manner. Gain hands on experience with real data from a variety of disciplines. The course will focus on the statistical computing language R.
Read moreBEM 392 -- WFU Spring 2024
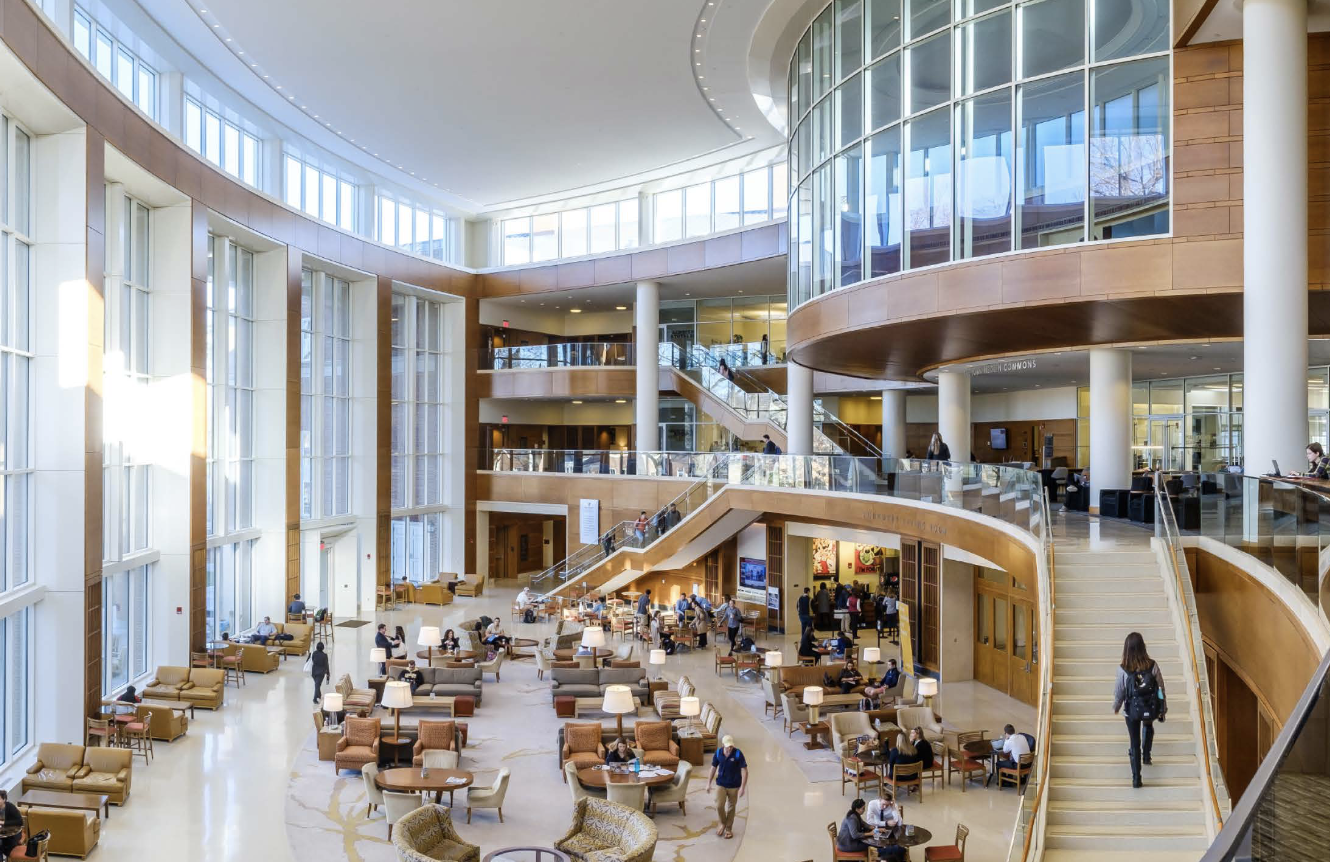
Seminar in Mathematical Business Analysis. The main purpose of this seminar is to develop the capability to apply quantitative knowledge to real and ill-defined problems. It tries to bridge the gap between the theory of quantitative decision approaches such as management science/operations research, information systems, and statistics (now mainly collected in the Business Analytics field), with the application of these approaches to the solution of actual business problems.
Read moreSTA 779 -- WFU Fall 2023
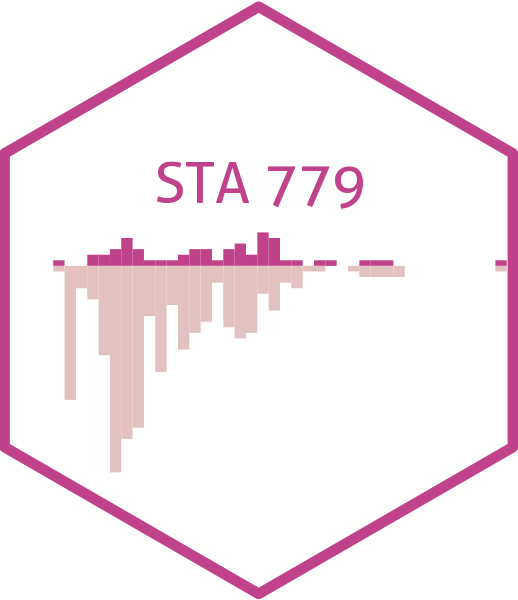
Causal Inference. From Correlation to Causation. The goal of this course is to give students the skills needed to conduct analyses and communicate results when causality is the goal. Students will learn how to implement causal inference techniques including matching and weighting, evaluate assumptions, and conduct sensitivity analyses.
Read moreWriting
Data Jamboree: A Party of Open-Source Software Solving Real-World Data Science Problems
The evolving focus in statistics and data science education highlights the growing importance of computing. This paper presents the Data Jamboree, a live event that combines computational methods with traditional statistical techniques to address real-world data science problems. Participants, ranging from novices to experienced users, followed workshop leaders in using open-source tools like Julia, Python, and R to perform tasks such as data cleaning, manipulation, and predictive modeling. The Jamboree showcased the educational benefits of working with open data, providing participants with practical, hands-on experience.
Read morePartnering with Authors to Enhance Reproducibility at JASA
The 'Why' behind including 'Y' in your imputation model
Missing data is a common challenge when analyzing epidemiological data, and imputation is often used to address this issue. Here, we investigate the scenario where a covariate used in an analysis has missingness and will be imputed. There are recommendations to include the outcome from the analysis model in the imputation model for missing covariates, but it is not necessarily clear if this recommendation always holds and why this is sometimes true.
Read more