Lucy D'Agostino McGowan
Assistant Professor of Statistics at Wake Forest University
Lucy helps answer public health questions using statistics. Her research focuses on causal inference, analytic design theory, and statistical communication.
Read More →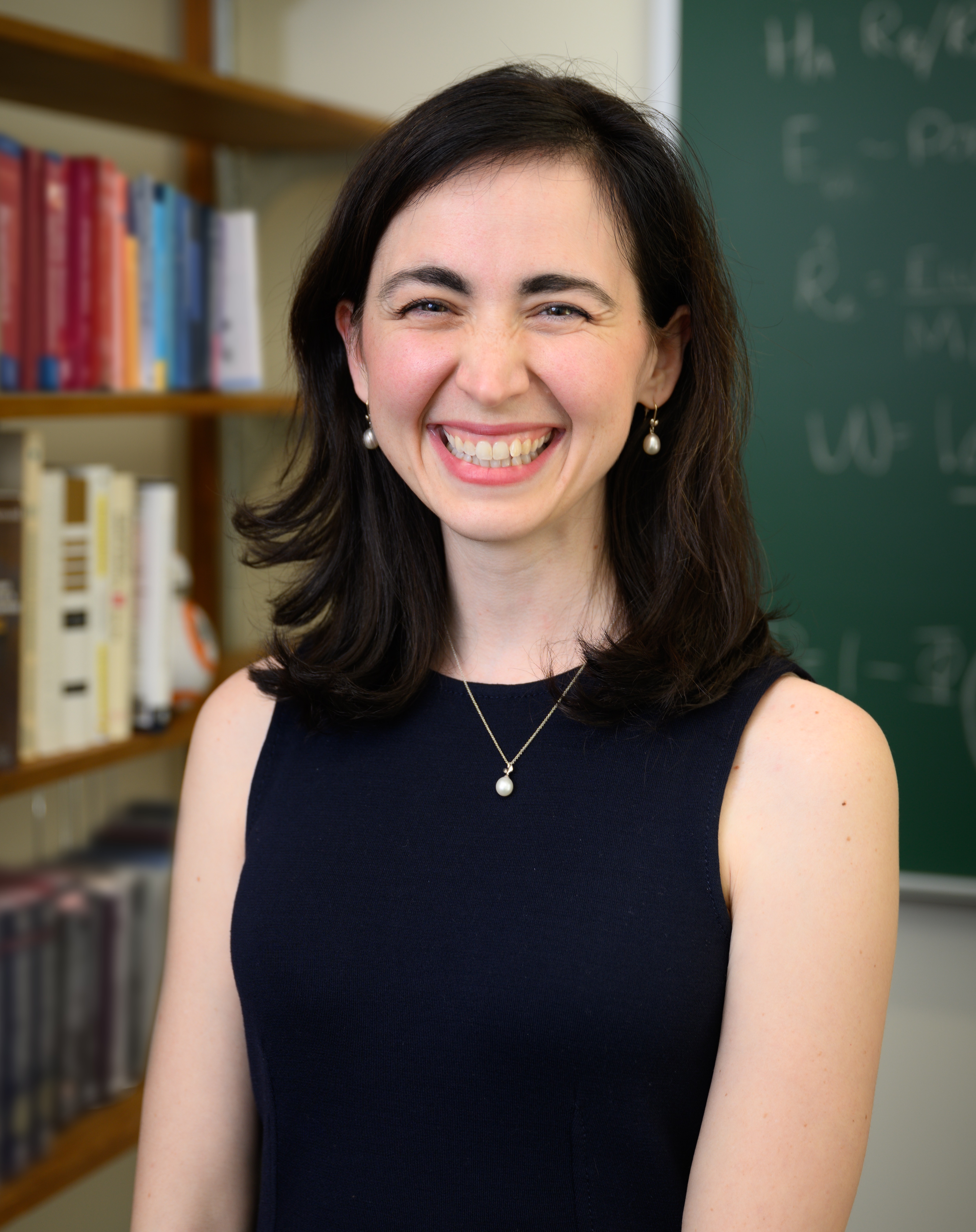